China's artificial intelligence (AI) industry has gone through several key milestones over the past decade. In 2017, Google's AlphaGo defeated the world champion of Go, Ke Jie, in China, confirming that AI had surpassed the highest level of human intelligence in a field once considered the pinnacle of human intellect. This event marked the beginning of a new explosive growth phase for the AI industry, with AI algorithms rapidly being implemented across various industries and generating value: from facial recognition and identity verification to algorithmic recommendations on internet platforms and targeted advertising. Throughout multiple technological cycles, a large number of companies that were initially affirmed and later validated have emerged.
SenseTime is one such company. Founded in 2014, SenseTime has reached its 10th year. From the earliest facial recognition algorithms to today's large-scale models, SenseTime has participated in and witnessed the development, challenges, and adjustments of China's AI industry. In December 2021, SenseTime went public on the Hong Kong Stock Exchange with a latest market value of 56.9 billion Hong Kong dollars. This year, SenseTime's market value has increased by more than 50%.
A decade has passed, and SenseTime has transformed from a rapidly growing AI star startup into a mature commercial company. It has experienced the fervor and subsequent calm in China's AI technology investment, two major transformations in AI technology, and the geopolitical impacts brought about by changes in the international landscape.
Advertisement
Today, the development of generative AI is progressing at an unprecedented pace, and the world is getting closer to the goal of general artificial intelligence. As a representative of China's AI industry, SenseTime is also quickly adjusting its strategic objectives. Unlike today's highly regarded startup newcomers, SenseTime, which has been in the AI field for ten years, is more focused on how to make a profit, how to truly implement AI technology, and how to become a technology company capable of weathering cycles.
A Decade of Struggle and Growth
SenseTime initially entered the AI industry through computer vision technology (CV). Xu Li, Chairman and CEO of SenseTime, recalls that when scientific breakthroughs entered the industry, everyone was verifying one thing: whether the precision of AI technology could pass the industrial red line. At that time, various companies were developing different AI models to test the waters in vertical industries. After about two to three years of verification, similar to AlphaGo's validation in Go, a batch of models centered on facial and image recognition passed the industrial application red line.
Around 2017, facial recognition technology began to be applied in various vertical scenarios, including smart cities and identity verification. Subsequently, the industry's new question was how to apply AI to more scenarios and industries. The more common approach at the time was to create more models.
Looking back today, the path of investing a large number of R&D personnel to create more domain-specific models has essentially been iterated. The mainstream approach is to create more general large-scale models and then fine-tune specialized models based on these models. At that node, few people thought about the further future or explored future paths, as the universality of the models still needed to be verified.Shangtang无疑是有远见的,率先投入通用模型的研发和AI算力的发展。"If you invest researchers to train different models for every scenario, you might end up with hundreds or thousands of models to complete a complex task, and the production of models will not come down." Xu Li found that the main cost of AI model production at this stage is human resources. In fact, at that time, the R&D costs of AI companies were almost equivalent to the salaries of R&D personnel.
As the commercialization process deepens, the development of general, end-to-end models has become the main direction of Shangtang's thinking. In 2019, Shangtang proposed to the outside world to develop a general visual large model; in the field of autonomous driving, it also took the lead in proposing to develop an end-to-end large model UniAD.
Xu Li found that in the process of moving towards generalization, the importance of AI computing power has increased. If a complex task can be divided into three sub-problems, assuming there are 10 parameters in each link, training 3 specialized models to solve these problems only requires 30 parameters, but if you want to use a general end-to-end model to solve it, it becomes 1000 parameters (10 times 10 times 10), which means the number of parameters has increased by 100 times, implying that the scale of computing power needs to increase exponentially.
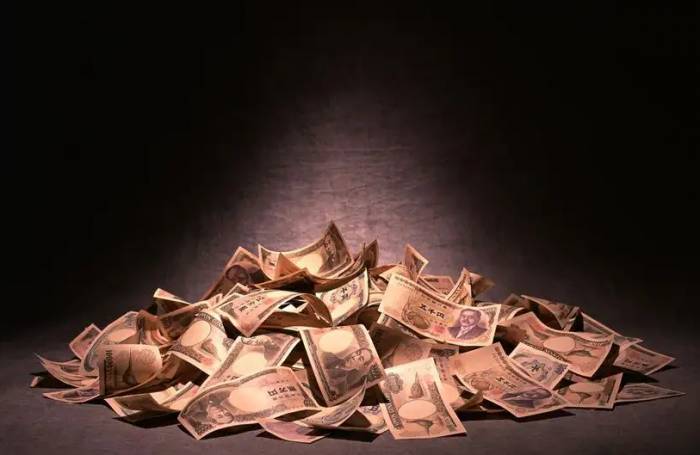
"If you use a different model for every scenario, you might end up with 1000 models, which is hard to achieve, and there are not enough people, and the price of models cannot come down." Xu Li found that this path is obviously getting further and further away from crossing the "industrial red line."
Therefore, the creation of general models became the top priority for Shangtang in 2019. Shangtang was one of the first batch of manufacturers in China to invest in the development of general large models, which also became its first-mover advantage in building an AI value commercial closed loop, and the large-scale AI infrastructure construction investment behind it was also put on the agenda. Xu Li originally planned to use rental computing power to complete the development of general models, but at that time there was no mature large-scale computing power infrastructure on the market, so he had to build it himself, not to mention that there was no previous experience in building a large-scale intelligent computing system. Shangtang became the first to eat crabs, investing in its own computing power large device, and forming a large device team to complete the construction of a super large-scale computing power training and pushing software platform. Naming the computing power large device, Xu Li compares its role in AI to that of a particle collider in high-energy physics. Now, Shangtang holds 54,000 GPUs and more than 20,000 petaFLOPS of computing power, which has become a scarce resource recognized by the industry.
Between 2018 and 2022, there have been huge changes in the attitude of capital towards AI and the international environment. From 2018 to 2021, it was a four-year period of explosive growth in China's AI entrepreneurship and financing, with AI startups represented by Shangtang quickly obtaining high financing and reaching the listing point in those years. Venture capital data service provider IT Orange shows that in 2018, China's AI field raised a total of 237.3 billion yuan, a year-on-year increase of 93%. In 2021, a total of 399.6 billion yuan was raised, a year-on-year increase of 51%.
However, the market热度slid明显下滑 in 2022, with AI financing falling to 157.9 billion yuan, a year-on-year decrease of 64%, and continued to decline to 110.1 billion yuan in 2023.
Along with the decline in financing enthusiasm, there were also changes in external water temperatures. Before this, AI field financing was mainly dominated by US investment institutions, which valued cutting-edge technology and were willing to pay for high R&D costs for startups in the early stage. However, in 2021, the US increased sanctions on Chinese technology companies, and US institutions gradually withdrew.
Shangtang's financing and listing process can be described as a microcosm: Shangtang was included in the US entity list in 2019, and during the listing period in 2021, it was also included in the US military-related enterprise list (CMIC). However, it still faced pressure and was listed on the Hong Kong Stock Exchange on December 30 of that year, obtaining a stable financing channel, enhancing risk resistance, which laid the foundation for Shangtang to continue to develop general intelligence and AI intelligent computing.
Like most AI companies in the world, Shangtang is still in the loss stage, but as a listed company, Shangtang faces the dual test of commercial landing and maintaining technological leadership. To turn losses into profits, it needs to increase revenue while reducing costs.It now appears that SenseTime's investment in AI infrastructure and its choice of a general-purpose large model approach align closely with international giants such as OpenAI. To excel in computational infrastructure and software capabilities, a deep understanding of large models is essential. In April 2023, SenseTime was the first in China to launch the "Riri Xin SenseNova" large model system. At the launch, Xu Li emphasized that the capabilities of a general model are more important than sheer scale. SenseTime has accumulated a significant number of clients over the years, addressing numerous industry issues, and thus has amassed a vast amount of real-world data, enabling the model to be more effective in vertical domains on top of its general utility. Within a year and three months following the launch, "Riri Xin" has evolved to version 5.5, with interactive effects and several core indicators achieving parity with GPT-4o, making it one of the leading domestic large models in China that benchmark against GPT-4 Turbo.
SenseTime's technological leadership has been swiftly reflected in its commercial success. The company's semi-annual financial report released in August this year shows that in the first half of 2024, SenseTime's revenue was 1.74 billion yuan, a year-on-year increase of 21%; gross profit was 770 million yuan, a year-on-year increase of 18%. In SenseTime's financial report, revenue sources are categorized into three main business areas: generative AI, intelligent vehicles, and traditional AI, with generative AI accounting for 60.4%, the highest proportion of SenseTime's revenue.
New Opportunities and Challenges
If we refer to the era of traditional AI, where models were built for specific scenarios, as AI 1.0, Xu Li believes that the most significant characteristic of the generative AI or AI 2.0 era, aside from the generality of the models, is the transformation of the cost structure from "research personnel-intensive" to "computational resource-intensive." OpenAI had only 87 researchers when developing ChatGPT. The ideal model is to support industry applications with a single set of computational infrastructure, achieving extremely low marginal service costs. However, in reality, the cost of computational resources is enormous, and with the current scale of applications, it is difficult to see a break-even point. In September 2024, Microsoft and BlackRock jointly established a $30 billion AI infrastructure fund.
SenseTime is one of the few AI companies that successfully went public during the last AI industry boom. Some industry insiders worry that SenseTime may not have the financial reserves of internet giants to massively invest in today's generative large models; nor can it, like startups, temporarily ignore commercial returns and obtain capital for technological investment through large-scale financing in the primary market.
Xu Li, however, believes that the past decade of AI 1.0 era customers and applications have given SenseTime a better understanding of what the market needs in terms of AI products and services. Secondly, due to its long-term focus on AI infrastructure and model applications, SenseTime has accumulated a considerable scale of computational power and technical resources, as well as the ability to operate these resources efficiently. "Electricity and communication traffic are both infrastructures; a single set of infrastructure can serve a thousand industries, but the early costs were also high. As technology iterates and the number of users expands, the marginal costs become negligible," and now AI infrastructure is at such an important turning point.
He concludes that SenseTime is the most model-savvy computational service provider and the most computationally adept model service provider.
SenseTime also needs to transform traditional AI clients into generative AI clients, allowing generative AI commercial applications to quickly enter the market as customer needs and technology iterate. In specific application industries, SenseTime needs to focus more. Industry clients from the AI 1.0 era have quickly deployed the Riri Xin large model across various fields with the advent of general artificial intelligence. Some vertical industry scenarios include the financial sector, where SenseTime's financial data pioneer product focuses on digital analysis; in the intelligent office sector, for example, SenseTime's "Raccoon" product, a personal AI assistant, has tens of thousands of personal users and developers and also serves top applications like Kingsoft Office. Additionally, in the field of large model human-like interaction, SenseTime's Riri Xin large model supports internet applications such as Sina Weibo, Yu Wen Group's Dream Island, and iQIYI, with daily token counts reaching tens of billions and the number of calls increasing nearly 22 times within half a year.
SenseTime's next product and business goals are "accessible, effective, and affordable." Accessibility means truly creating value for users; effectiveness means integrating into customers' production and processes; affordability means significantly reducing the costs of training, inference, and deployment.
To achieve this goal, SenseTime's decision-making layer has formulated a trinity core strategy of "large-scale facilities - large models - applications." Large-scale facilities refer to the infrastructure services of the model, primarily computational services. Xu Li mentioned that if there are only infrastructure services without an understanding of large models, there is no competitiveness. Today, the main uses of computational power are twofold: training models and using models. When training models, it is necessary to optimize the efficiency of computational power usage; when using models, it is necessary to save on computational power costs."Today, the business models of artificial intelligence, whether it's training models or using models to provide external services, essentially consume resources and pay for those resources. All business models ultimately equate to the consumption of computing resources, which is achieved through a 'trinity' to utilize resources in the most effective way," said Xu Li.
Following such strategic layout, SenseTime believes that the deep collaboration between computing power and models can enable rapid iteration of large models and reduce inference costs, thereby gaining more users and increasing the number of calls, which leads to increased revenue. For example, in inference scenarios, SenseTime has achieved a fourfold increase in the number of requests per second (QPS) under the same computing power and electricity costs through innovative technical architecture, and has realized the elastic scaling of inference services on demand, optimizing the overall cost of large-scale AI inference.
Computing power services are also a key direction for many tech giants, and Xu Li believes that the focus of some tech giants is on their own ecosystem, including self-developed chips and cloud platforms. However, in the current AI field, to seize the initiative, one should use whatever resources are faster and better, not limited to a single company's products and platforms. Xu Li believes that the basic services provided by SenseTime are closer to the current state of AI development.
Two Legs to Cross the Technology Cycle
Today, the biggest challenge in the AI field is the unclear business model. The investment in computing power, data, and talent are relatively certain, and even the risks and threats that AI may bring have been discussed many times. However, how AI can make money and what the final product form will be are still difficult to conclude.
Whether an AI technology company like SenseTime can successfully cross the new round of technology transformation cycles depends on two points: first, moving fast enough; second, moving far enough.
Xu Li said that today's challenges for AI companies are significant because "technology investment will always be ahead of commercialization." If one decides to work on large models, it requires long-term resource investment and keeping up with new directions. But the actual returns may take a long cycle to materialize.
After going public, SenseTime has a clearer plan - profitability.
Xu Li said that SenseTime currently has two legs, one leg is traditional AI, with mature technology, continuously reducing costs, expanding markets (including overseas markets), and focusing on profit contribution; the other leg is the new generation of AI large models, aiming for break-even, this leg grows quickly and has a foreseeable future. The former ensures that SenseTime "moves fast enough," and the latter ensures "moves far enough."
SenseTime is constantly exploring different business models, such as selling integrated machines. A machine with a certain number of accounts can achieve localized deployment, buy and use immediately, which on the one hand can reduce the threshold for customers to apply AI, and on the other hand, it can also help customers save on usage costs. This model has a relatively low gross profit compared to the software model, but the commercialization efficiency is significantly improved.In overseas markets, SenseTime has not abandoned the software model. In fact, globally, China and the United States are the two highlands in the field of AI, and Chinese AI technology is very advanced for many foreign countries. Xu Li said that overseas customers have a high willingness to purchase AI software, and the payment cycle is short. Currently, SenseTime already has very mature products and solutions in the traditional AI field, and only needs to continue to expand overseas sales channels.
It is understood that SenseTime's current annual revenue growth rate in overseas markets is about 40%, higher than the overall growth rate of 21%, and the proportion of overseas markets in the group's total revenue has increased to 18.5%. At present, the overseas market is one of the main sources of profit for SenseTime, and it can continue to use the software model in overseas markets, with higher gross margins. The growth of revenue and profits in overseas markets can also help SenseTime better invest in large model businesses.
Next, the test for AI companies is how to achieve the commercialization of large models. According to media reports, the training cost of OpenAI GPT-4 is about $63 million, and the total training cost in 2022 is about $540 million. OpenAI said that the operating cost in 2024 will exceed $8.5 billion, with an expected loss of about $5 billion, and the total loss (excluding equity compensation) from 2023 to 2028 is expected to reach $44 billion. OpenAI also mentioned that the cost of model training will continue to increase, and it is expected to reach $9.5 billion per year by 2026.
It can be predicted that large models will continue to move forward on the general path, but it is difficult to achieve true generalization in a short time. In addition, as the capabilities of open-source models become stronger, it is not very valuable for some technology companies to "roll" parameters and do pre-training. A more practical way is to improve the specialized capabilities of smaller models through targeted training, which is also equivalent to "reducing costs and increasing efficiency". This may be a more market-oriented approach.
From training to inference, the resource attribute of computing power is becoming more and more apparent. SenseTime is also actively deploying computing power operations, hoping to monetize the resources that have been invested under current conditions. The reality is that the computing power resources in the market are currently scattered and the standards are not unified, making the efficiency of use not high. SenseTime can provide computing power operation services, connecting cards with different standards, adapting to different needs, and meeting the needs of customers who need to use computing power.
On October 18, 2024, at the 10th anniversary international forum of SenseTime Technology, Xu Li said that we are currently at the turning point of AGI (General AI), and the rapid development of large models is largely due to the significant improvement in infrastructure levels, making general AI models possible. Xu Li also mentioned that as early as 2014, when SenseTime was established, SenseTime founder Tang Xiaoou emphasized bringing technology into daily life, hoping that technology can be integrated into different scenes of life. This sentence is still not outdated today.